In today’s highly competitive market, understanding consumer preferences is no longer optional, it’s essential. But how do businesses determine what features, prices, or benefits truly drive consumer decisions? Enter conjoint analysis, a powerful and versatile research methodology that provides actionable insights into consumer decision-making.
This comprehensive guide will explore conjoint analysis in detail, its value, different types, working mechanisms, real-world examples, and how tools like Decode can support and enhance the process.
What is Conjoint Analysis?
Conjoint analysis is a statistical technique used to measure consumer preferences for different attributes of a product or service. By analyzing the trade-offs consumers are willing to make, it uncovers the relative importance of each attribute and helps businesses prioritize them accordingly.
Key Concept of Conjoint Analysis
The idea behind conjoint analysis is simple: consumers evaluate a product or service as a bundle of attributes rather than in isolation. For instance, when buying a car, they consider factors like fuel efficiency, brand reputation, price, and design together, not separately.
How it Works in Practice
Respondents are presented with a series of choices, each representing a unique combination of attributes. Their choices reveal how much they value individual features, enabling companies to align their offerings with consumer preferences.
Why is Conjoint Analysis Important?
Conjoint analysis is widely used across industries because it bridges the gap between what consumers say they want and what they are willing to choose. Here are some reasons why it is indispensable:
1. Accurate Consumer Insights
- Understand what drives purchasing decisions.
- Identify trade-offs consumers are willing to make between price and features.
2. Optimized Product Development
- Focus on attributes that matter most to your target audience.
- Save resources by eliminating less-valued features.
3. Improved Pricing Strategies
- Determine how sensitive consumers are to pricing changes.
- Set optimal price points that maximize revenue while maintaining demand.
4. Market Segmentation
- Identify distinct consumer segments based on their preferences.
- Tailor marketing and product strategies to meet segment-specific needs.
5. Scenario Simulation
- Predict market response to new products or attribute changes.
- Test hypothetical scenarios to reduce risks.
Types of Conjoint Analysis
Conjoint analysis is a versatile technique with multiple methodologies tailored to specific needs. Each type has its strengths and ideal use cases:
.png)
1. Choice-Based Conjoint Analysis (CBC)
- The most widely used form.
- Respondents select their preferred product from sets of options.
- Ideal for simulating real-world purchasing behavior.
2. Adaptive Conjoint Analysis (ACA)
- Dynamically adjusts questions based on previous responses.
- Focuses on attributes most relevant to the respondent.
- Suitable for complex products with numerous attributes.
3. Full-Profile Conjoint Analysis
- Respondents evaluate products with all attributes simultaneously.
- Provides holistic insights but can be overwhelming with many attributes.
4. Partial-Profile Conjoint Analysis
- Only a subset of attributes is presented in each choice task.
- Reduces cognitive load while still capturing key trade-offs.
5. MaxDiff Analysis (Maximum Difference Scaling)
- Participants identify the most and least important attributes in each set.
- Great for ranking features or understanding attribute importance.
6. Menu-Based Conjoint Analysis:
- Respondents build their ideal product by selecting options from a "menu."
- Ideal for customizable offerings like meal plans or tech devices.
Step-by-Step Conjoint Analysis Process
Conducting a conjoint analysis involves several critical steps, each designed to extract meaningful insights from consumer choices.
Step 1: Define Attributes and Levels
Identify the product attributes (e.g., price, size, color) and their levels (e.g., $10, $20; small, medium, large). This forms the foundation of your study.
Step 2: Design the Experiment
Use experimental design techniques to create a set of product profiles or scenarios that combine the attributes and levels in a structured way.
Step 3: Survey Creation
Develop a user-friendly survey that presents respondents with these profiles, asking them to make choices or rank preferences.
Step 4: Data Collection
Distribute the survey to a representative sample of your target audience. Ensure the sample size is adequate to derive statistically significant insights.
Step 5: Data Analysis
Use specialized software to analyze the responses. Techniques like regression analysis or hierarchical Bayes estimation are often used to calculate:
- Part-Worth Utilities: The value consumers assign to each attribute level.
- Importance Scores: The relative importance of each attribute.
Step 6: Generate Insights and Simulate Scenarios
Interpret the results to make informed decisions about product features, pricing, or market positioning. Scenario simulation helps in testing hypothetical product configurations.
How Does Conjoint Analysis Work?
Conjoint analysis breaks a product or service into attributes and their levels, combining them in different ways to explore customer preferences. This approach mimics real-world decisions by asking respondents to select from thoughtfully designed product options.
Hypothetical Example: Choosing a Laptop
Imagine a conjoint study on laptops. The product is divided into these attributes:
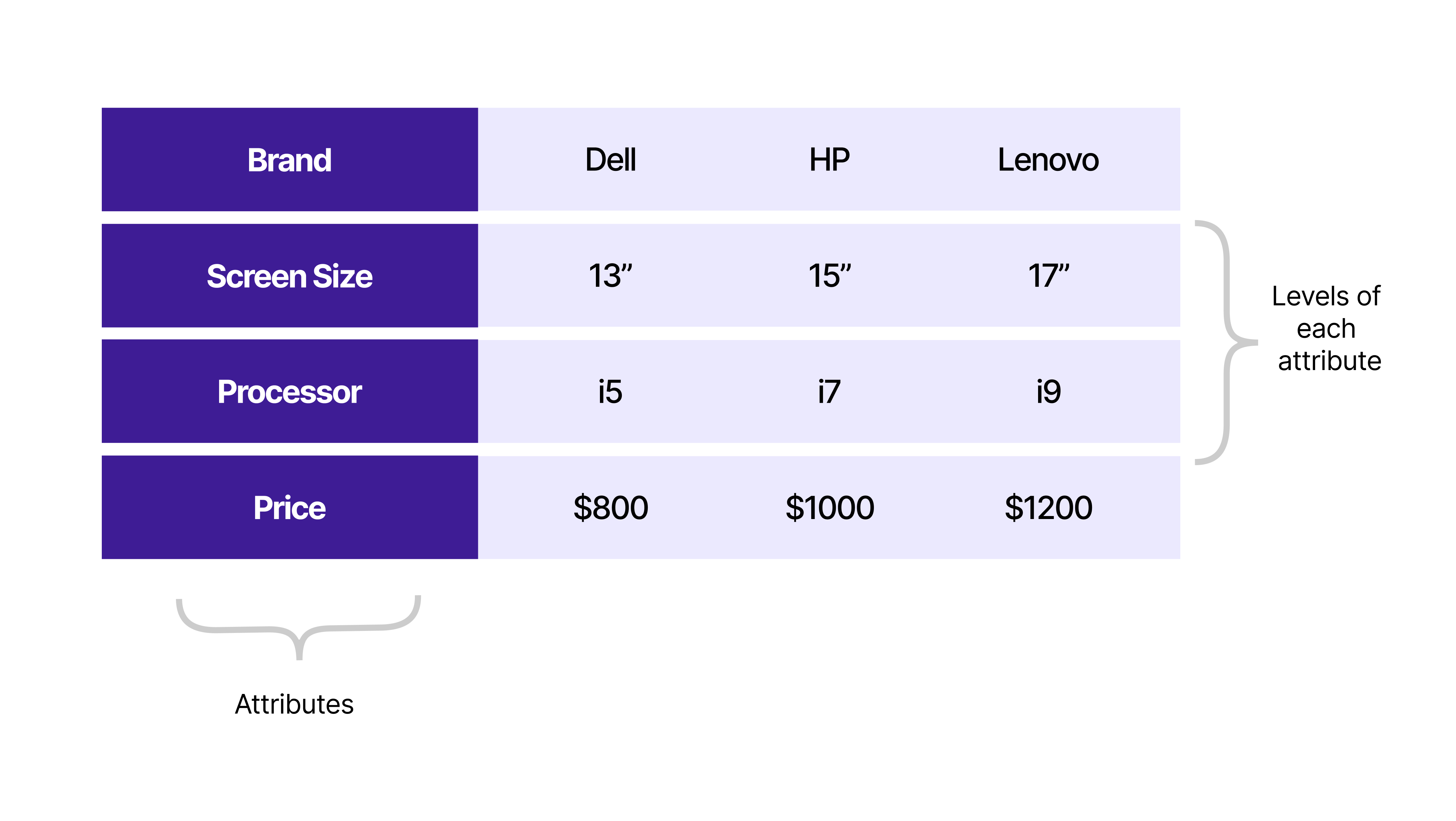
Each choice task presents the respondent with unique combinations of attributes, like this:
Example Conjoint Choice Task
Which of these laptops would you buy?
Respondent Action: Select one option.

Understanding Preference Scores in Conjoint Analysis
In conjoint analysis, customer preferences are quantified using preference scores, also known as partworth utilities or utility scores. These scores measure the influence of each attribute and its levels on respondents’ choices. They provide a numerical representation of how much value consumers assign to different product features.
Hypothetical Example: Preference Scores for a Laptop
Using survey results, preference scores are calculated for each level within the attributes. For instance:
- Brand: Dell (+30), HP (+20), Lenovo (+10)
- Screen Size: 13" (+10), 15" (+15), 17" (+30)
- Processor: i5 (+10), i7 (+20), i9 (+30)
- Price: $800 (+50), $1,000 (+20), $1,200 (+0)
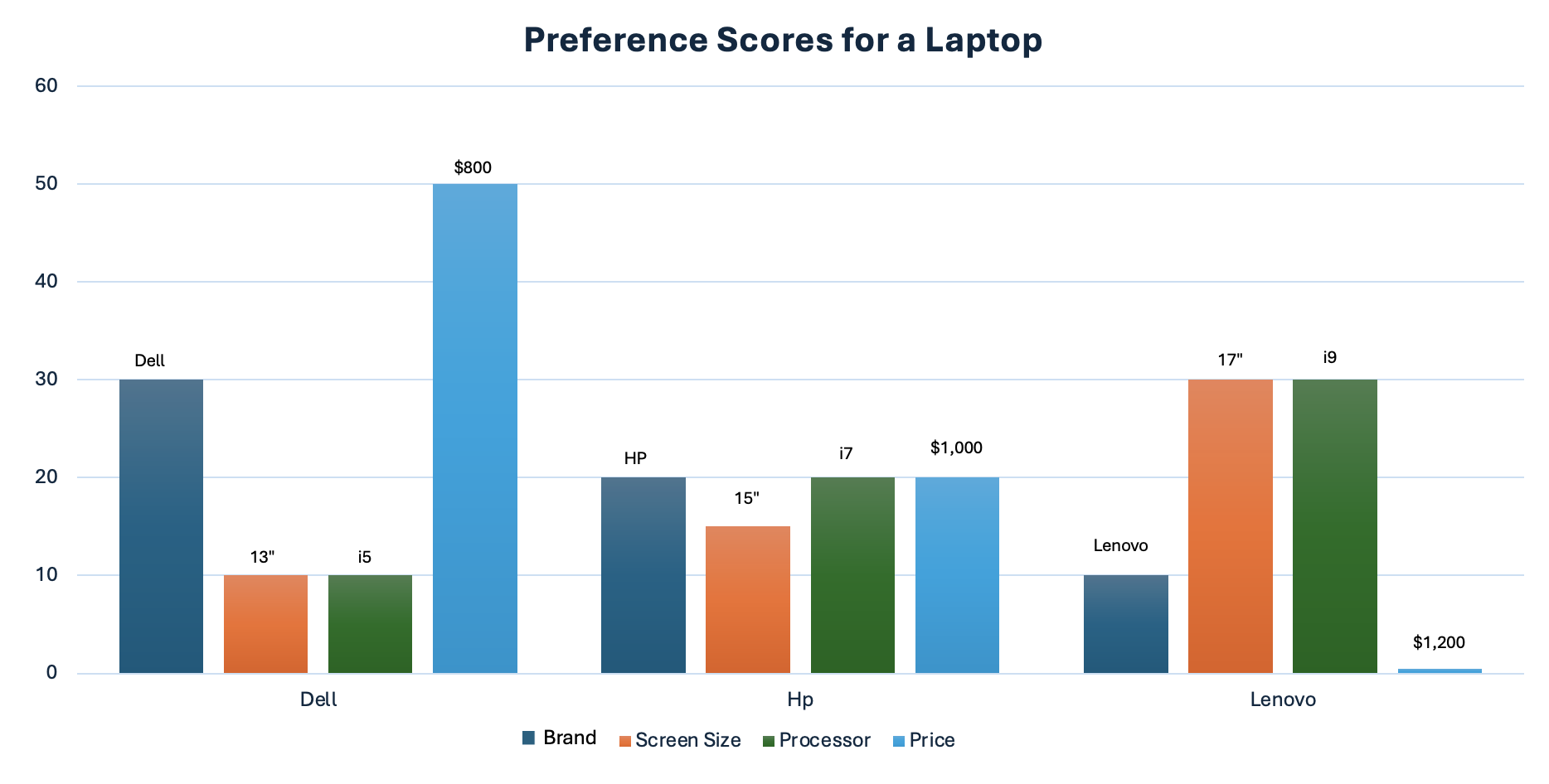
The higher the preference score, the more appealing that feature is to respondents.
From Preference Scores to Market Insights
Preference scores are not just standalone numbers, they are used to build market simulators, tools that predict customer behavior in real-world scenarios. These simulators allow businesses to:
- Estimate Market Share: Simulate the percentage of customers likely to choose a specific product.
- Test Feature Sensitivity: Understand how small changes (e.g., lower prices or better processors) affect customer preferences.
- Balance Value and Cost: Identify optimal product combinations that deliver value to customers while remaining cost-effective for the company.
Example Market Simulation
Using preference scores, a company can simulate market outcomes for different laptop configurations:

Simulations like these help companies refine product offerings and develop pricing strategies tailored to market demand.
Why Preference Scores Matter
Preference scores empower businesses to create customer-focused products by highlighting the most valuable features and their ideal combinations. By leveraging these insights, companies can make data-driven decisions to optimize product design, pricing, and market positioning.
Conjoint Algorithm: How It Works
Conjoint analysis is a powerful tool used to understand consumer preferences by determining the perceived value of different product or service features. It helps businesses make informed decisions about product design, pricing strategies, and market positioning by simulating consumer choices based on various attributes. The algorithm behind conjoint analysis helps calculate the utility values (also known as part-worths) of each attribute level, allowing businesses to understand what drives consumer decisions.
At its core, we use a logic model paired with the Nelder-Mead Simplex algorithm to estimate these utility values. The advantage of this method is that it allows for a cohesive and comprehensive survey experience, all within a single platform. This enables businesses to run sophisticated analyses without having to rely on multiple separate tools or platforms.
Let’s break down how the conjoint algorithm works:
Step 1: Understanding the Model’s Structure
To explain the calculations involved, we use the following notations:
- R: The total number of respondents.
- T: The number of tasks presented to each respondent.
- C: The number of concepts within each task.
- A: The number of attributes involved in the study.
- L: The number of levels within each attribute.
For each attribute, we define a part-worth value w′(a,l)w′(a,l), which represents the utility of a specific attribute level llfor attribute aa. The total number of part-worths is represented by w(s)w(s), a one-dimensional array consisting of part-worths for all levels of all attributes.
Step 2: Constructing the Experimental Design
Each respondent is presented with a set of concepts, each defined by a combination of attribute levels. These concepts are represented in a four-dimensional matrix XX, where each entry XrtcXrtc corresponds to the availability (1 or 0) of a specific attribute level in a concept for a given task and respondent.
- X is a matrix of size R×T×C×SR×T×C×S, where SS is the total number of attribute-level combinations.
- Y is the binary outcome matrix, where each element YrtcYrtc is 1 if respondent rr selects concept cc in task tt, and 0 otherwise.
Step 3: Utility Calculation for Each Concept
The utility of a concept, UxUx, is the sum of the part-worths for the attribute levels that are present in the concept. This is calculated by taking the scalar product of the concept’s attributes vector x(s)x(s) and the part-worths vector w(s)w(s). The formula for calculating the utility of concept xx is:
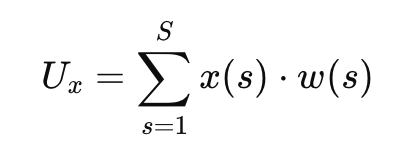
Where:
- x(s)x(s) is 1 if attribute-level combination ss is present in the concept, otherwise 0.
- w(s)w(s) is the corresponding part-worth for attribute-level combination ss.
Step 4: Using the Multinomial Logit (MNL) Model
In the multinomial logit model (MNL), the probability of a respondent choosing a particular concept depends on the utilities of all concepts in the task. For example, if there are two concepts 11 and 22, the probability that concept 1 is chosen is given by the following equation:

For a choice between more than two concepts, the probability of selecting concept cc is:
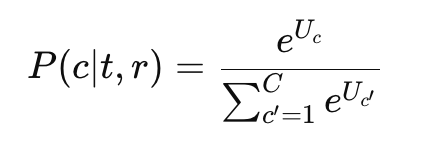
Where:
- UcUc is the utility of concept cc,
- CC is the total number of concepts in task tt.
Step 5: Log-Likelihood Measure
The Log-Likelihood (LL) measure is used to estimate the fit of the model. It is calculated by comparing the predicted probabilities (from the MNL model) to the observed choices made by respondents. The Log-Likelihood function is given by:

The goal is to find the part-worth vector ww that maximizes this Log-Likelihood.
Step 6: Solving for Part-Worths Using Maximum Likelihood
To estimate the part-worths, we use Maximum Likelihood Estimation (MLE), which seeks the part-worth vector wwthat maximizes the Log-Likelihood function. This involves solving a nonlinear continuous maximization problem that requires optimization techniques.
We use the Nelder-Mead Simplex Algorithm to optimize this function. This algorithm iteratively adjusts the values of the part-worths to maximize the Log-Likelihood. The process involves:
- Specifying initial guesses for the part-worths, often starting at zero.
- Iterating through adjustments to these values to maximize the Log-Likelihood function.
Step 7: Rescaling the Part-Worths
Once the part-worths are estimated, they are rescaled so that the part-worths for each attribute across its levels have a mean of zero. This is done by subtracting the mean part-worth for each attribute from all levels within that attribute:

This step ensures that the part-worths are comparable across attributes, facilitating interpretation and decision-making.
Applications of Conjoint Analysis
1. Pricing Strategy in Retail
A retail company uses conjoint analysis to understand how price impacts customer decisions for a new line of smartwatches. Insights reveal the price elasticity, enabling the company to set a price that maximizes revenue without losing demand.
2. Product Development in the Automotive Industry
An automaker conducts a conjoint study to determine the trade-offs consumers are willing to make between fuel efficiency, luxury features, and price. The results guide the design of a new SUV tailored to consumer priorities.
3. Marketing Strategy for Subscription Services
A streaming service employs conjoint analysis to evaluate the importance of features like ad-free content, offline downloads, and subscription price. This helps in creating tiered plans that appeal to different customer segments.
4. Customization in Food Delivery
A food delivery app uses menu-based conjoint analysis to optimize customizable meal plans. By understanding which options are most valued, the app enhances user satisfaction and increases orders.
Advanced Techniques in Conjoint Analysis
1. Hierarchical Bayes Estimation: A robust statistical method for estimating individual-level preferences. It is widely used in choice-based conjoint analysis to account for variability across respondents.
2. Latent Class Analysis: Segments the market into distinct groups based on shared preferences. This helps in targeted marketing and product development.
3. Conjoint Simulator Tools: Interactive simulators allow businesses to test different scenarios, such as changing an attribute or introducing a new competitor, to predict market behavior.
Challenges in Conjoint Analysis
Despite its effectiveness, conjoint analysis has limitations:
- Complexity in Design: Poorly designed surveys can overwhelm respondents or fail to capture meaningful insights.
- Data Interpretation: Requires expertise in statistical methods to derive actionable results.
- Cognitive Load: Respondents may struggle with too many attributes or complex choices, affecting the quality of data.
- External Factors: Real-world decisions may be influenced by external factors not captured in the study, such as brand loyalty or social influence.
How Decode Supports Conjoint Analysis
Decode, an AI-powered customer insights platform, revolutionizes conjoint analysis by combining cutting-edge technology with user-friendly tools.
1. Seamless Survey Creation
- Simplified tools for defining attributes and levels.
- Pre-built templates for various industries and use cases.
2. AI-Driven Data Analysis
- Advanced algorithms provide faster and more accurate insights.
- Automated part-worth utility calculations and importance scores.
3. Predictive Scenario Simulations
- Test hypothetical product configurations or pricing changes.
- Forecast market responses with high accuracy.
4. Real-Time Dashboards
- Interactive visualizations make it easy to interpret data.
- Shareable reports streamline communication with stakeholders.
5. Integration Capabilities
- Connects seamlessly with existing CRM, ERP, or survey platforms.
- Ensures smooth data collection and analysis workflows.
6. Expert Support
Decode’s team of research professionals offers tailored guidance, from survey design to data interpretation, ensuring maximum ROI.
Conclusion
Conjoint analysis is a game-changing methodology that empowers businesses to decode consumer preferences with unparalleled precision. From optimizing product features and pricing to crafting targeted marketing strategies, its applications are vast and impactful.
Whether you're a marketer, product manager, or researcher, leveraging conjoint analysis can unlock valuable insights to drive smarter decisions. With advanced tools like Decode, the process becomes even more accessible, enabling you to transform data into actionable intelligence effortlessly.
Invest in conjoint analysis today, and stay ahead in the race to understand and meet consumer demands.