Imagine being able to test your website, app, or service without ever needing to recruit real participants, yet still gathering critical insights into user behavior. This futuristic-sounding process is made possible by synthetic users - simulated personas that mimic human actions on digital platforms. As user expectations for flawless digital experiences rise, synthetic users are reshaping how companies ensure their platforms meet these demands. The way we conduct product research is evolving, and synthetic users are leading the charge. These AI-generated participants offer exciting new possibilities but also come with unique challenges. In this blog, we’ll explore synthetic users, their benefits, limitations, and how they’re shaping the future of research.
What Are Synthetic Users?
Synthetic users refer to simulated personas or bots that are programmed to mimic human behavior and interaction with digital interfaces. These users do not represent actual customers but serve as a tool for testing, monitoring, and improving various digital functionalities. By creating synthetic users, companies can conduct controlled and measurable experiments to evaluate system performance, identify bugs, or optimize customer journeys.
The creation of synthetic users typically involves complex algorithms designed to simulate specific user behaviors such as clicking on links, navigating pages, or completing transactions. These simulated users provide actionable insights into how real users might interact with a website or application, thus helping in diagnosing pain points and enhancing the user experience.
How do Synthetic Users Work?
The foundation of synthetic users lies in advanced algorithms and machine learning models, specifically designed to replicate human actions on digital platforms. Here’s a closer look at how this powerful product functions:
.png)
- Behavioral Data Analysis: Synthetic users are built on behavioral data collected from real user interactions. This data is analyzed to identify typical user actions, preferences, and patterns. By examining this data in detail, machine learning models can create accurate simulations of human behavior.
- Generative Models: GANs and VAEs: Two prominent methods in generating synthetic users are Generative Adversarial Networks (GANs) and Variational Autoencoders (VAEs):
- GANs consist of two neural networks that work in tandem to generate and refine data. One network creates , while the other evaluates it, ensuring the final output closely resembles real user behavior.
- VAEs work by learning from real user interactions to generate data based on the distribution patterns observed. This makes the synthetic user actions feel natural and aligned with realistic user scenarios.
- User Persona Simulation: Synthetic users are programmed to mimic specific user personas, replicating behaviors like clicking, scrolling, or abandoning a cart. These personas range from occasional visitors to power users, enabling platforms to evaluate how various types of users interact with their website or application.
- Testing and Real-Time Monitoring: Once created, synthetic users are deployed on digital platforms to interact in ways that mimic genuine user behavior. This testing phase identifies performance issues, monitors user experience, and can even reveal potential security vulnerabilities through simulated malicious actions.
- Integration with Existing Testing Infrastructure: Synthetic user products are often built to integrate seamlessly with existing analytics and testing tools. This allows businesses to view synthetic user interactions alongside real user data, providing a comprehensive view of platform performance and user journey bottlenecks.
Through this complex setup, synthetic users empower businesses with insights that would otherwise require significant time and resources, accelerating the optimization of digital experiences.
How to Create Effective Synthetic Users
Designing synthetic users requires an in-depth understanding of user behavior and the platform’s core functionality. The key to creating effective synthetic users is to make them as realistic as possible. This involves simulating different user personas, from casual visitors to power users, who will each interact with your platform in unique ways. Here’s a quick guide to the key steps:
- Behavior Mapping: Start by identifying key actions real users take on the platform, like navigating, clicking, or making transactions. This mapping serves as the foundation for programming synthetic behaviors.
- Data-Driven Modeling: Use real user data to train algorithms, ensuring synthetic users emulate common behaviors. Machine learning techniques like Generative Adversarial Networks (GANs) and Variational Autoencoders (VAEs) are useful for capturing natural patterns.
- Diverse User Profiles: Develop multiple synthetic personas to simulate varied user demographics. Include different preferences, device types, and usage behaviors for a more comprehensive view of platform interaction.
- Realistic Timeframes and Patterns: Mimic realistic interaction patterns, such as time on page, click speed, or bounce rate. This prevents synthetic data from appearing artificial and skewing results.
- Testing and Refinement: Continuously test and refine synthetic users to ensure they align closely with real user behavior. Adjust behaviors as platform or user patterns evolve.
Why Are Synthetic Users Important?
The importance of synthetic users lies in their ability to test digital products without involving real human participants, making them a cost-effective and efficient alternative for continuous performance monitoring. Their usage has surged, especially in sectors like e-commerce, SaaS, and web development where flawless UX is critical for success.
Key Benefits of Using Synthetic Users
- Scalability: Synthetic users can be created in large numbers, allowing companies to simulate massive traffic loads to stress-test platforms before going live.
- Cost Efficiency: Unlike traditional usability tests, synthetic users eliminate the need for physical testers, saving both time and money.
- Repeatability: Testing with synthetic users can be repeated under the same conditions, ensuring consistency in performance metrics.
- Risk Mitigation: These bots can simulate malicious activities like hacking attempts or data breaches, allowing companies to identify vulnerabilities and secure their platforms.
Applications of Synthetic Users
1. Website Load Testing
One of the primary applications of synthetic users is load testing. Before launching a website or major updates, it is crucial to ensure that the platform can handle large volumes of traffic. Synthetic users can be deployed to simulate thousands of visitors interacting with the website simultaneously. This enables businesses to observe how their servers handle stress, pinpoint bottlenecks, and fine-tune performance before real customers encounter any issues.
2. E-commerce Conversion Optimization
For e-commerce platforms, conversion rate optimization (CRO) is essential. Synthetic users can simulate the buyer’s journey, from landing on a product page to completing a purchase. By analyzing synthetic user behavior, companies can identify which stages of the sales funnel might cause friction and lead to cart abandonment. This data is invaluable in making strategic adjustments to improve conversion rates and maximize revenue.
3. Fraud Detection and Security Testing
Synthetic users are widely used in fraud detection and cybersecurity testing. For example, banks and financial institutions can deploy synthetic users to simulate fraudulent activities, like unauthorized transactions, to test the robustness of their security protocols. Similarly, websites can simulate malicious bots attempting DDoS attacks, helping businesses safeguard their digital assets and protect sensitive user data.
4. UX Testing and User Journey Optimization
User experience is a critical factor that affects engagement, retention, and conversion. By simulating user interactions, businesses can collect valuable data on how users navigate through their platforms. This helps in refining the user journey by identifying any pain points, slow-loading pages, or confusing interfaces that could negatively affect real users.
5. Monitoring and Troubleshooting
Synthetic users can be deployed 24/7 to monitor system performance and identify issues in real-time. For instance, if a certain feature on a website is malfunctioning, synthetic users can instantly report these errors, allowing businesses to troubleshoot problems proactively. This helps maintain the seamless operation of websites and applications, ensuring a smooth experience for real users.
6. Financial Services
Financial platforms must prioritize security, ease of use, and trust. Synthetic users help simulate account management, payments, and fraud detection systems, ensuring a seamless user experience while adhering to stringent privacy standards.
7. Gaming
In the gaming industry, synthetic users are used to test gameplay mechanics, interface usability, and player engagement. These simulations help developers tweak game dynamics to enhance user enjoyment and retention.
Synthetic Users: Game-Changing Innovation or Threat to Authentic Research?
Synthetic users represent a breakthrough in digital testing and research, offering undeniable benefits but also introducing risks that could fundamentally alter how we understand user experience and behavior. Let’s dive into the debate surrounding synthetic users: are they a cost-saving innovation, or could their widespread adoption signal a troubling shift in the accuracy and ethics of research?
1. The Case for Synthetic Users as a Cost-Saving Solution
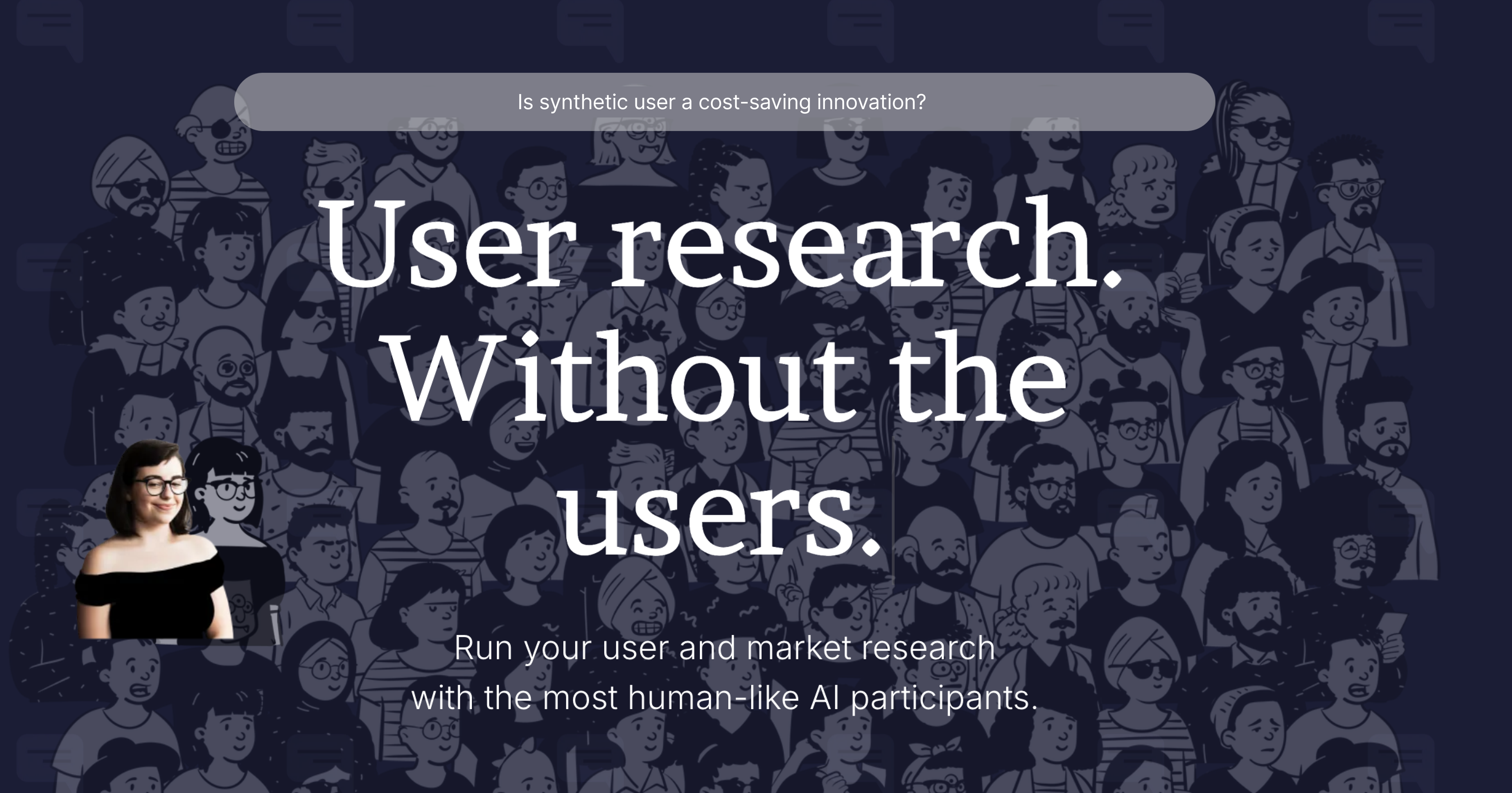
Synthetic users offer several practical advantages that make them appealing to businesses and researchers alike, particularly in terms of cost efficiency and scalability.
- Elimination of Recruitment Costs: Traditional user testing requires recruiting, scheduling, and compensating participants, which is time-consuming and costly. Synthetic users bypass these logistical challenges by providing a ready-to-use testing base.
- Scalable Testing at Low Cost: With synthetic users, companies can simulate massive numbers of interactions and user journeys on a platform. This allows for extensive testing without exponential cost increases, offering significant financial and operational savings.
- Continuous Monitoring and Real-Time Feedback: Synthetic users can run 24/7, providing constant feedback on a platform’s performance, which would be impractical and expensive with human testers. This round-the-clock monitoring is critical for industries that need immediate issue detection and correction, such as e-commerce, gaming, and finance.
2. The Potential Downside: Research Apocalypse?
While synthetic users have promising applications, they also pose challenges and ethical concerns that, if unchecked, could disrupt the accuracy and integrity of research and testing.
- Lack of Genuine Human Emotion and Nuance: Synthetic users may accurately simulate behaviors like clicks and navigation, but they cannot replicate the complex emotional and cognitive processes that drive real human decisions. As a result, companies might miss out on insights related to emotional engagement, satisfaction, and brand perception.
- Risk of Biased Data: Since synthetic users are created from algorithmic interpretations of past data, they may inadvertently perpetuate biases inherent in the original dataset. For example, if a model is trained on a dataset that over represents certain demographics, synthetic users could mimic behaviors that don’t reflect a broader audience, leading to skewed insights.
- Over-Reliance and Replacement of Real Testing: Some companies may be tempted to use synthetic users as a replacement for real user testing to save costs, but this could result in shallow insights that lack the depth of real human experiences. Certain experiences and reactions - especially those involving emotional responses - require real human engagement to capture.
3. Finding a Balance: Synthetic Users as a Complement, Not a Replacement
Instead of viewing synthetic users as a replacement for human testing, they are perhaps best seen as a complementary tool that can support and enhance real user research. Combining synthetic and real user data allows businesses to gain a fuller picture: synthetic users provide volume and speed, while human testers bring depth and nuance.
- Combining Real and Synthetic Insights: For robust research, a mix of synthetic and real user testing can offer both scalability and emotional insights. This balanced approach helps in uncovering both technical issues and emotional drivers.
- Selective Use Cases: For instance, synthetic users are ideal for stress-testing, performance monitoring, and quick feedback. Meanwhile, real users are critical for testing UI/UX elements that depend on emotional engagement and complex decision-making.
Real User Interview vs Synthetic User Interview
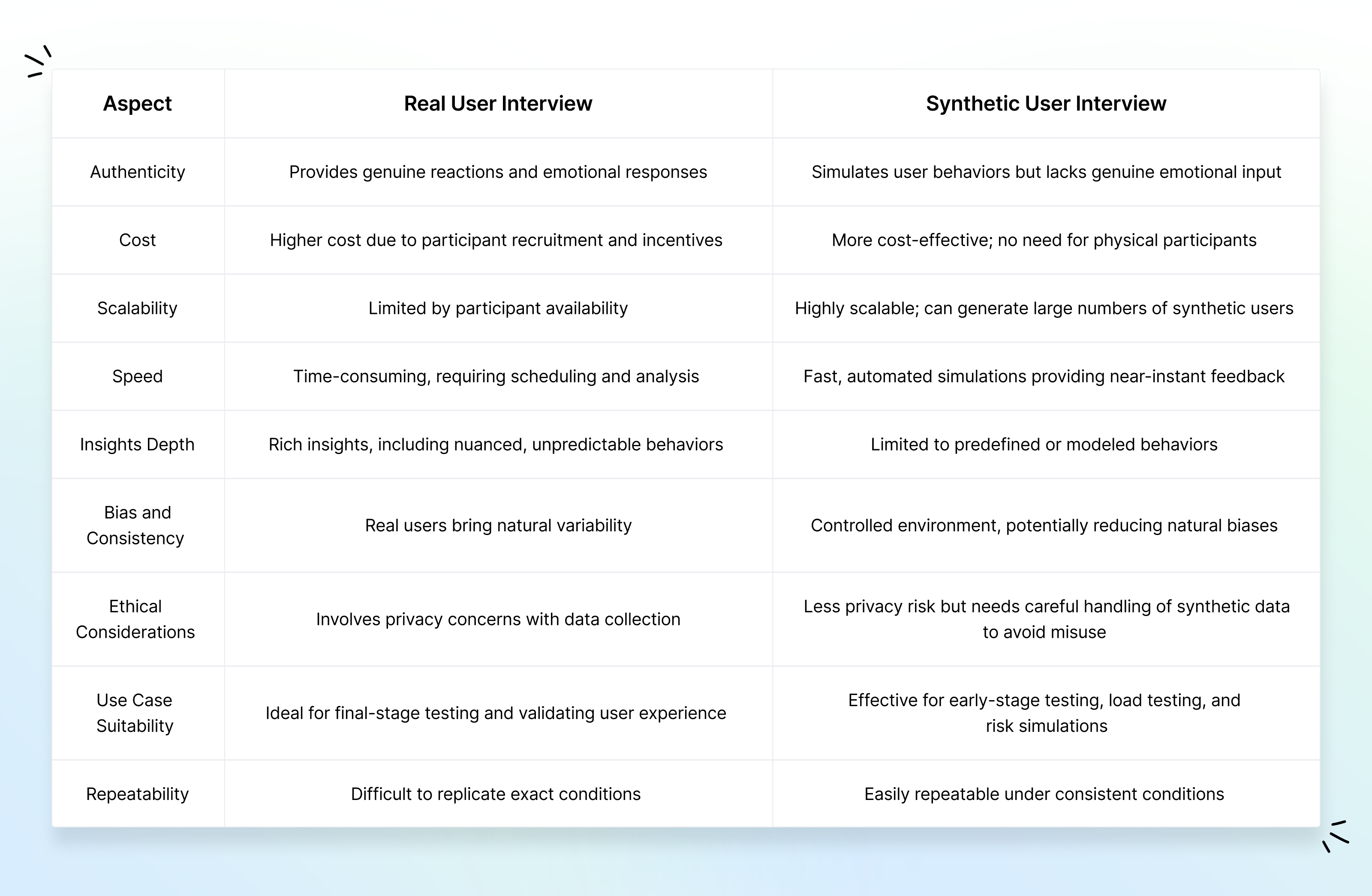
Challenges and Ethical Considerations
Despite the numerous advantages of using synthetic users, there are challenges and ethical considerations that businesses need to be aware of. The use of bots, if mismanaged, can lead to skewed data, which may result in poor decision-making. Ensuring that synthetic users are programmed to replicate real-world scenarios as closely as possible is essential for obtaining accurate insights.
Potential Ethical Concerns
- Data Privacy: While synthetic users do not represent real individuals, companies must ensure that they do not misuse the data collected from interactions with synthetic users. Transparency about data use is essential.
- False Data Infiltration: Synthetic users should not interfere with real users or disrupt analytics by creating fake traffic. Measures must be taken to separate real user data from synthetic user interactions.
Integrating Synthetic Users into Existing Research Processes
Incorporating synthetic users into your current research workflow can provide valuable, data-driven insights without the need for continuous, costly user recruitment. Here’s how to make the most of synthetic users in your research process:
- Clarify Study Objectives: Start by defining the primary objectives and questions you want to answer. Are you aiming to test site navigation, optimize conversion funnels, or improve overall user experience? Having clear objectives helps you design synthetic user scenarios that align with these goals.
- Embed Synthetic Users in Testing: Once you’ve established your goals, begin incorporating synthetic users to test various aspects of your platform. These AI-driven personas can run usability tests, simulate user journeys, and help identify common friction points. Synthetic data helps in making informed decisions about product design, layout adjustments, and functionality improvements.
- Leverage Data to Enhance User Experience: The data gathered from synthetic users reveals how different user types interact with your product, surfacing preferences, trends, and potential pain points. Use these insights to refine the user experience, whether by adjusting design elements, simplifying navigation, or personalizing features based on synthetic behavioral patterns.
- Optimize Continuously: Integration doesn’t end with initial findings. Continuously refine and iterate based on new synthetic user data to adapt to evolving user needs. By regularly evaluating performance metrics, you can keep improving usability and streamline the product experience for real users.
- Cost-Effective Testing: Synthetic users reduce the need for extensive real-user testing, saving time and resources. With automated testing at scale, you get consistent and repeatable data that can simplify decision-making and streamline your testing processes.
How to Use Synthetic User Findings Responsibly
While synthetic users offer efficiency and scalability, responsibly interpreting their findings is essential to avoid misleading insights. Here’s how to leverage synthetic data effectively:
- Recognize Limitations: Understand that synthetic findings lack real human emotions and contextual nuances. Avoid making final decisions based solely on synthetic user data.
- Complement with Real User Data: Use synthetic insights as a foundation, but always validate them with real user testing when possible, especially for UX and emotional response testing.
- Define Clear Boundaries: Reserve synthetic testing for tasks like load, security, or A/B testing. Limit the scope of synthetic users to scenarios where real user insights are not critical.
- Separate Data Streams: Keep synthetic and real user data distinct to prevent inflated metrics or misinterpretation in analytics and performance reports.
- Adhere to Ethical Standards: Ensure transparency about synthetic user usage in reporting, and avoid misleading stakeholders by clearly labeling synthetic data in presentations and reports.
Is Synthetic Research a Better Alternative to No Research?
The debate surrounding the value of synthetic research versus real user research has been prominent in the UX community. When a team lacks the resources, skills, or access to a user population, synthetic users can provide some level of insight into user behaviors and preferences. While this method may offer a semblance of understanding, the risk lies in the inaccuracies and misrepresentations that can arise from relying solely on synthetic data. Without the corrective lens of real-user insights, teams may make misguided design or business decisions, investing significant time and resources into strategies that ultimately miss the mark.
Moreover, there is a danger that teams may become overly reliant on synthetic research, potentially stalling their progression toward conducting genuine user research. When stakeholders see value in synthetic insights for minimal investment, justifying the need for comprehensive user studies can become a challenge. This reliance can foster a false sense of security, leading to incorrect conclusions that, when uncovered, could undermine the organization's understanding of the critical role of user-centered research. Much like the debate over AI-generated companions, while synthetic interactions may fulfill an immediate need, they could also hinder the development of authentic human connections-both in interpersonal relationships and in user experience design. Ultimately, a balanced approach that incorporates both synthetic and real-user research is essential for fostering a deeper and more accurate understanding of users.
Conclusion
In a world where digital performance is crucial for business success, synthetic users provide an innovative solution for testing, optimizing, and securing online platforms. Their ability to mimic real-world behavior at scale allows businesses to anticipate potential challenges, improve user experiences, and safeguard their systems from fraud and cyber threats. By harnessing the power of synthetic users, companies can stay ahead of the curve, delivering flawless digital experiences that drive engagement and conversion.