Imagine knowing which products your customers will buy next, anticipating market shifts before they happen, or identifying risks before they escalate. Predictive analytics makes all this possible, providing businesses with a window into the future based on data from the past and present. With advancements in artificial intelligence (AI), machine learning (ML), and big data, predictive analytics is rapidly transforming the way businesses operate.
In this blog, we will explore predictive analytics in depth—what it is, how it works, its core components, real-world applications, and how it can be applied in various industries to unlock untapped opportunities for growth and efficiency.
What is Predictive Analytics?
Predictive analytics is a branch of advanced analytics that uses statistical techniques, data mining, and machine learning to analyze historical data and make predictions about future outcomes. At its core, predictive analytics goes beyond just understanding what happened in the past—it allows businesses to forecast what is likely to happen in the future.
The Foundation of Predictive Analytics
Predictive analytics rests on three pillars
- Historical Data: Data from past events is analyzed to detect trends and patterns. This data could come from various sources, such as transactional databases, CRM systems, social media, or IoT devices.
- Statistical Algorithms: These are used to interpret the data and create models that help forecast future outcomes.
- Machine Learning (ML): Algorithms learn from the data and become more accurate over time. This allows models to continuously improve and adapt to new data.
For instance, if you run an online retail store, predictive analytics can analyze past customer purchases, their browsing history, and behavioral data to predict which products they are likely to buy next. This allows you to offer personalized recommendations or anticipate which items to stock up on before a major shopping season.
Why Predictive Analytics Matters?
Predictive analytics is more than just a trend; it’s a critical component of modern business strategy. Here’s why it matters:
- Proactive Decision-Making: Businesses can move from reactive to proactive strategies, taking actions before problems arise or opportunities slip away.
- Improved Customer Insights: With predictive analytics, businesses can personalize customer experiences, anticipate needs, and offer tailored solutions that enhance engagement.
- Operational Efficiency: Companies can streamline their supply chain and inventory management, ensuring they have the right products at the right time.
- Risk Mitigation: Predictive models help identify potential risks, such as fraud in banking or equipment failure in manufacturing, before they become costly issues.
How Does Predictive Analytics Create Value?
The core value of predictive analytics is its ability to enable proactive decision-making. Businesses can leverage it to anticipate customer behavior, optimize resources, and identify risks before they happen. Some examples include:
- Retailers forecasting product demand during specific seasons.
- Banks detecting potential fraudulent activities.
- Healthcare providers predicting patient outcomes and improving care.
By harnessing predictive analytics, businesses can stay ahead of the curve, shaping strategies that drive growth and efficiency.
Key Types of Business Analytics
Predictive analytics is one of four key branches of business analytics, each serving a different purpose in understanding and improving business outcomes:
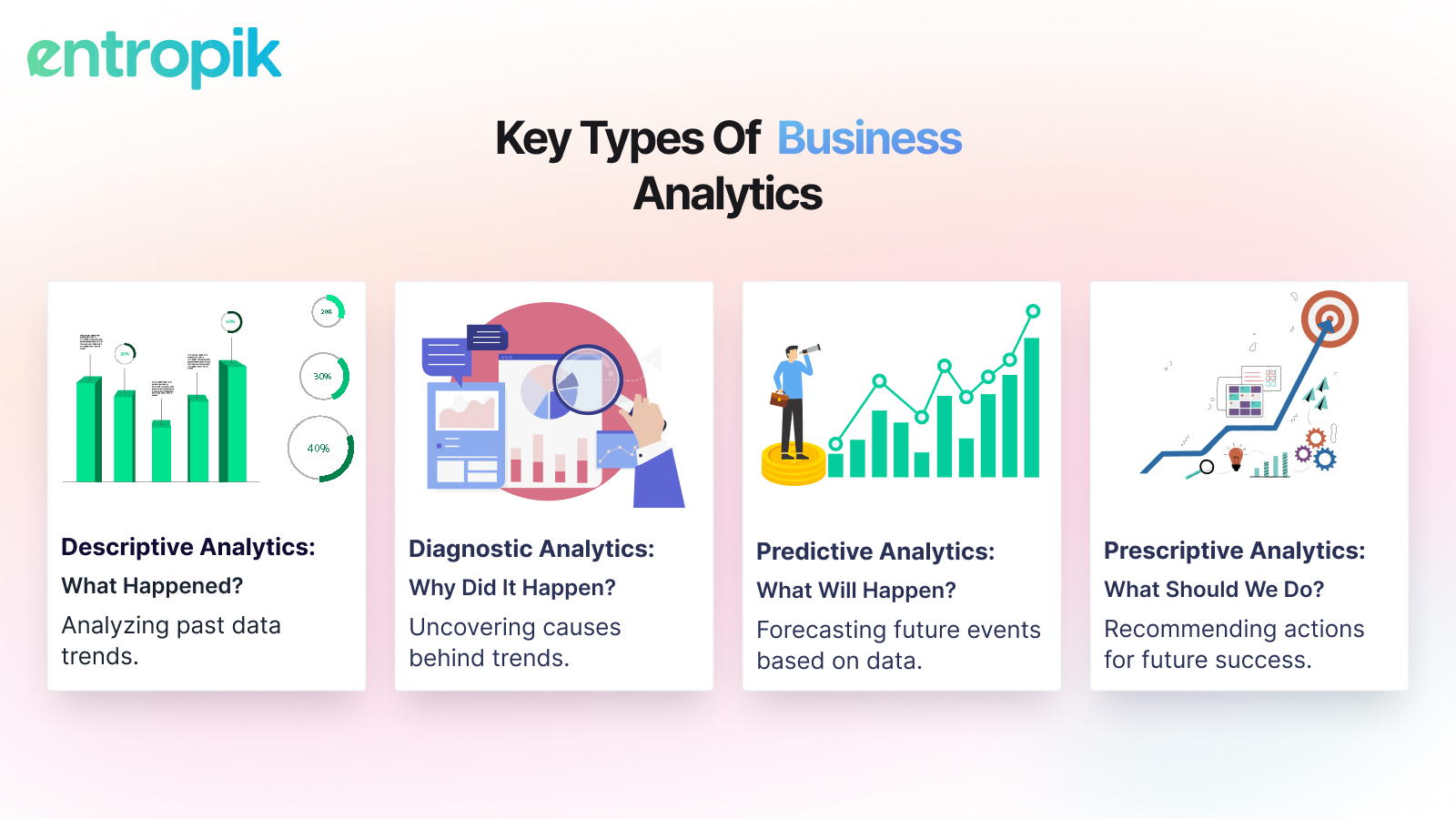
1. Descriptive Analytics: What Happened?
- Descriptive analytics focuses on summarizing past data to explain what has happened. It helps businesses understand historical performance, such as sales trends, customer demographics, or website traffic.
- Tools like dashboards, reports, and data visualizations (e.g., graphs, charts) are often used for descriptive analytics. For instance, a retailer might use descriptive analytics to track which products were top sellers over the past year.
2. Diagnostic Analytics: Why Did It Happen?
- Diagnostic analytics goes beyond describing what happened; it answers why certain outcomes occurred. By drilling down into the data, businesses can identify the root causes of specific trends or issues.
- For example, if a company's sales decline in a particular region, diagnostic analytics might reveal that a competitor’s aggressive pricing strategy was responsible for the drop.
3. Predictive Analytics: What Will Happen?
- This branch focuses on forecasting future trends and outcomes based on historical patterns. Predictive models allow businesses to plan for future scenarios and make proactive decisions to optimize results.
- For example, airlines use predictive analytics to forecast demand for flights and adjust ticket prices in real-time based on expected customer behavior.
4. Prescriptive Analytics: What Should We Do?
- Prescriptive analytics suggests specific actions to achieve desired outcomes. It takes predictions one step further by recommending the best course of action based on the data.
- In the financial sector, for example, prescriptive analytics might recommend an optimal investment portfolio based on predicted market conditions.
Predictive Analytics – A Human Trait?
From ancient civilizations trying to predict weather patterns for successful harvests to modern-day stock traders using data to anticipate market shifts, humans have long tried to predict future events. In essence, predictive thinking is hardwired into human decision-making. When faced with a choice, we rely on past experiences to estimate outcomes and make informed decisions—whether it’s buying a product, choosing a career path, or crossing the street.
Predictive analytics formalizes this human trait by using vast amounts of data and sophisticated algorithms. The difference is that, while humans can intuitively predict outcomes based on a limited set of information, machines can process millions of data points and identify patterns that the human brain cannot. In doing so, predictive analytics brings unprecedented precision and scale to the art of prediction, turning a deeply human instinct into a powerful business tool.
How Does Predictive Analytics Work?
At a high level, predictive analytics works by identifying patterns in historical data and using those patterns to make informed predictions about future events. Let’s break down the process:

1. Data Collection
The first step in any predictive analytics project is gathering relevant data. This could include:
- Transactional data from databases (e.g., sales history, CRM data).
- Behavioral data (e.g., customer browsing patterns).
- External data (e.g., social media sentiment, weather data, economic indicators).
2. Data Preparation
Once data is collected, it needs to be cleaned and organized. This step ensures that the data is accurate and complete. Tasks in this phase include removing duplicates, filling in missing data, and formatting the data for analysis.
3. Model Selection
Different types of predictive models are selected depending on the problem at hand. Common models include:
- Regression Models: Used to predict a continuous outcome (e.g., sales revenue) based on the relationships between variables (e.g., marketing spend, seasonality).
- Classification Models: Classify data into categories (e.g., whether a customer will churn or stay).
- Decision Trees: Visual models that represent different decision paths and their possible outcomes.
- Time Series Models: Used for forecasting based on historical data trends over time.
4. Model Training and Testing
Once a model is selected, it is "trained" using historical data. This allows the model to learn patterns within the data. After training, the model is tested against new data to assess its accuracy.
5. Prediction Generation
Once the model is validated, it is used to generate predictions based on new or real-time data. For example, a retailer might use a predictive model to forecast demand for specific products during an upcoming holiday season.
6. Monitoring and Refinement
Predictive models require continuous monitoring to ensure they remain accurate as new data becomes available. If a model’s predictions start to deviate from actual outcomes, it must be refined and updated.
Key Techniques Used in Predictive Analytics

Predictive analytics employs a range of advanced techniques to derive insights from data:
- Regression Analysis: This technique explores relationships between variables. For instance, how does an increase in marketing spend affect sales? Linear regression can be used to quantify the relationship between these variables.
- Classification Models: These models group data into predefined categories. For instance, a telecom company might classify customers as likely or unlikely to churn based on their usage patterns.
- Decision Trees: Decision trees map out possible outcomes based on different choices. They are easy to interpret and commonly used in scenarios like customer segmentation or risk assessment.
- Neural Networks: These models are inspired by the human brain and are highly effective for complex problems like image recognition or detecting fraud in financial transactions.
- Clustering: Clustering groups similar data points together, often used for market segmentation. This allows companies to target different customer groups with tailored marketing campaigns.
- Time Series Analysis: Time series analysis forecasts future values based on historical data trends. It is commonly used in sales forecasting, stock market predictions, or demand planning.
Uses and Examples of Predictive Analytics
Predictive analytics can be applied across a wide range of industries, offering businesses the ability to solve complex challenges:
1. Retail:
- Personalized Recommendations: Retailers like Amazon use predictive models to recommend products to customers based on their past behavior.
- Demand Forecasting: Predictive analytics helps retailers optimize their inventory by forecasting demand for specific products, ensuring they stock up on items that will sell and reduce overstock.
2. Healthcare:
- Patient Outcomes: Predictive models can assess the likelihood of a patient being readmitted based on their health records, allowing providers to take preventive measures.
- Disease Outbreaks: Public health agencies use predictive analytics to anticipate disease outbreaks, enabling timely interventions.
3. Finance:
- Fraud Detection: Financial institutions use predictive models to detect fraudulent transactions by analyzing patterns in customer behavior.
- Credit Scoring: Banks and lenders can predict the likelihood of a customer defaulting on a loan by analyzing their financial history and other risk factors.
4. Manufacturing:
- Predictive Maintenance: Manufacturers use predictive analytics to predict when machinery is likely to fail, allowing for proactive maintenance and minimizing downtime.
- Supply Chain Optimization: By predicting demand fluctuations, manufacturers can adjust their production and supply chain operations to optimize costs and resources.
Benefits of Predictive Analytics in Commercial Business
The benefits of predictive analytics for businesses are transformative. Here are some key advantages:
1. Enhanced Decision-Making
Predictive analytics equips businesses with data-driven insights that enable more accurate and confident decision-making. Whether forecasting demand, optimizing pricing, or predicting customer churn, predictive analytics helps businesses stay ahead of the curve.
2. Increased Efficiency
By predicting trends and future demand, businesses can streamline their operations and minimize waste. For example, manufacturers can optimize inventory and reduce production costs, while retailers can improve supply chain efficiency.
3. Improved Customer Retention
Predictive models can identify early signals of customer churn, allowing businesses to intervene with targeted marketing campaigns or retention strategies. This is especially critical for subscription-based services, where customer lifetime value is directly tied to retention rates.
4. Risk Mitigation
Businesses can identify potential risks before they escalate. Whether it's predicting equipment failure in manufacturing or identifying potential loan defaults in finance, predictive analytics helps businesses reduce costly risks and improve long-term outcomes.
5. Competitive Advantage
Companies that successfully implement predictive analytics gain a competitive edge by understanding market trends, consumer preferences, and operational efficiencies. By anticipating changes before they occur, businesses can adapt faster than their competitors.
Conclusion: Shaping the Future with Predictive Analytics
Predictive analytics is no longer just a tool for data scientists. With the right platform and approach, it can become a powerful asset for any business, guiding decisions that drive growth, efficiency, and innovation. As predictive models become more advanced and accessible, companies that leverage this technology will be well-positioned to stay ahead in an increasingly data-driven world.
If you're looking to explore the full potential of predictive analytics, platforms like Decode provide an accessible, AI-powered way to start predicting the future and optimizing your business strategy today.
Frequently Asked Questions (FAQs) about Predictive Analytics
Q1. What is predictive analytics used for?
A: Predictive analytics is used to forecast future events and trends, helping businesses make data-driven decisions.
Q2. Which industries benefit most from predictive analytics?
A: Industries like finance, healthcare, retail, and manufacturing benefit the most from predictive analytics.
Q3. What tools are popular for predictive analytics?
A: Tools like IBM SPSS, Microsoft Azure Machine Learning, and SAS are commonly used in predictive analytics.
Q4. What are the challenges in implementing predictive analytics?
A: Challenges include data quality issues, ethical concerns, model complexity, and integration with existing systems.
Q5. How can businesses get started with predictive analytics?
A: Businesses should start by building a strategy, choosing the right tools, and focusing on data quality and model accuracy.
Predictive Analytics with Decode
Decode, Entropik’s comprehensive AI-powered insights platform, supports businesses in harnessing the power of predictive analytics. With Decode, companies can effortlessly apply predictive analytics to consumer behavior and market data to uncover actionable insights. Here’s how Decode makes predictive analytics easier and more impactful:
- Advanced Predictive Models: Decode offers pre-built predictive models customized for user behavior, marketing effectiveness, and product success. These models provide actionable insights across industries like CPG, retail, and technology.
- Behavioral and Emotional Data: Decode leverages Emotion AI, eye tracking, and facial coding to predict consumer behavior, giving businesses deeper insights into how their target audience will respond to campaigns, products, and experiences.
- Real-time Data Integration: Decode ensures access to real-time data, enabling businesses to continuously refine and improve their predictive models based on the latest information.
- Ease of Use: Decode’s intuitive interface allows non-technical users to apply predictive models without requiring deep expertise in data science, democratizing access to predictive insights.
{{cta-button}}