Imagine you’re tracking your daily coffee consumption and your productivity at work. You notice that on days when you drink more coffee, you also seem to accomplish more tasks. It’s tempting to conclude that drinking coffee directly boosts your productivity right? However, a closer look reveals that the real culprit could be the demanding projects you have on those days, leading you to consume more coffee to stay alert. This scenario exemplifies the difference between correlation and causation.
In research and data analysis, understanding these concepts is crucial. While correlation shows a statistical relationship between two variables, causation indicates that one variable directly affects another. Misinterpreting these terms can lead to incorrect conclusions and poor decision-making, making it essential to grasp the distinctions between them.
What is Correlation?
Correlation refers to a statistical relationship between two variables, indicating how they change together. When two variables are correlated, it means that when one variable increases, the other tends to either increase or decrease. However, correlation does not imply that one variable causes the change in the other.
Types of Correlation:
1. Positive Correlation: Both variables move in the same direction. For example, as the temperature increases, ice cream sales also rise.
2. Negative Correlation: The variables move in opposite directions. For instance, as the number of hours spent studying increases, the number of errors on a test may decrease.
3. Zero Correlation: No relationship exists between the variables, indicating that changes in one do not affect the other.
Read more: Correlation Research explained in Detail
What is Causation?
Causation, on the other hand, implies a direct cause-and-effect relationship between two variables. When one variable causes a change in another, we say there is causation. Establishing causation is more complex than identifying correlation and typically requires controlled experiments or longitudinal studies.
Key Criteria for Causation:
1. Temporal Precedence: The cause must occur before the effect.
2. Covariation: The two variables must be correlated.
3. No Alternative Explanations: Other potential factors should be ruled out.
Correlation vs Causation: What’s the Difference?
- Nature of Relationship: Correlation indicates a relationship between variables, while causation indicates a direct cause-and-effect link.
- Statistical Analysis: Correlation can be identified through statistical measures like the Pearson correlation coefficient, while causation requires more rigorous methods, such as experiments.
- Misinterpretation Risks: Assuming causation from correlation can lead to faulty conclusions, such as attributing a rise in coffee consumption to increased productivity without considering other factors (e.g., workload).
For instance, in a recent analysis of a streaming service, researchers discovered a correlation between the number of playlists created by users and their overall engagement time on the platform. As users created more playlists, their total viewing time also increased.
While this indicates a relationship between playlist creation and increased engagement, it does not imply that creating playlists causes users to watch more content. Other factors, such as the variety of available content or user interest levels, could also contribute to both increased playlist creation and higher engagement.
This is a classic example of correlation whereas, conversely, consider a social media app that implemented a new feature allowing users to customize their profiles with background images. A/B testing was conducted to measure the impact of this feature on user retention rates.
The results showed that users who utilized the profile customization feature had a retention rate of 30% higher than those who did not. In this case, the introduction of the new feature directly led to increased user retention, establishing a clear cause-and-effect relationship. The temporal sequence of the feature’s implementation and the observed increase in retention confirm this causation.
In UX research, understanding the distinction between correlation and causation is vital for drawing accurate conclusions that inform design and strategy decisions. These concepts play a crucial role in interpreting user data and shaping the overall user experience.
Correlation in UX Research
For instance, consider a mobile application that tracks fitness activity. A UX researcher might find a correlation between users who engage with community features (like forums or social sharing) and their overall app usage time. This observation suggests that users who are more socially engaged also tend to spend more time in the app.
While this correlation provides valuable insights, it is important to remember that correlation does not imply causation. In this case, we cannot definitively conclude that increased community engagement causes longer app usage. Other factors could contribute to both variables, such as the user’s interest in fitness, motivation from peers, or the app’s design features that encourage interaction. This is a classic example of how correlation can highlight potential relationships but doesn’t provide a clear direction for action.
Importance of Identifying Correlation:
Identifying correlation is useful in the exploratory phase of UX research. It helps researchers spot trends and areas for further investigation. By understanding which variables are correlated, UX teams can formulate hypotheses for deeper study. However, it’s crucial to approach correlated data with caution, ensuring that findings do not lead to hasty conclusions or misguided design changes.
Methods for Identifying Correlation
There are various methods to identify correlations in UX research:
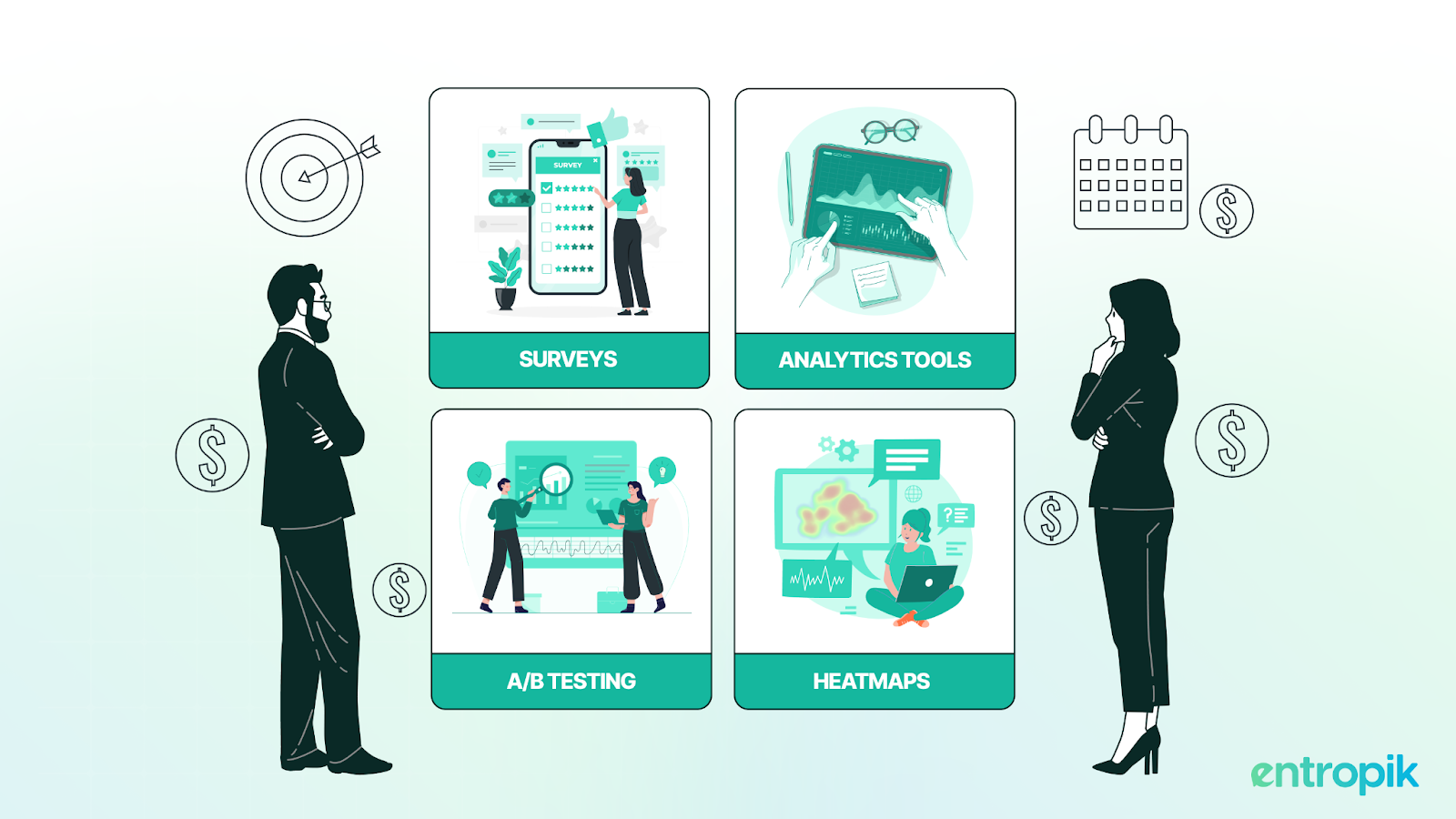
- Surveys and Questionnaires:
- Collecting user feedback through surveys can reveal correlations between user satisfaction and specific features or functionalities. For instance, if users report high satisfaction scores alongside frequent use of a particular feature, researchers can explore this relationship further.
- Analytics Tools:
- Utilizing web analytics tools like Google Analytics can help track user behavior and identify correlations. For example, tracking metrics such as page views, session duration, and bounce rates can uncover relationships that inform website design decisions.
- A/B Testing:
- A/B testing allows researchers to compare two or more versions of a product to see how changes affect user behavior. By analyzing data from different user groups, researchers can identify correlations between changes made (like color, layout, or functionality) and user engagement.
- Heatmaps:
- Heatmaps visualize user interactions on a webpage, showing where users click, scroll, and hover. By analyzing heatmap data, UX researchers can correlate user behavior with design elements, providing insights into which features are most engaging.
Read more: Top A/B testing tools you should know
Causation in UX Research
In contrast, causation denotes a direct cause-and-effect relationship between variables, suggesting that one variable directly influences another. Establishing causation typically requires more rigorous research methodologies, such as controlled experiments or longitudinal studies.
For example, if a company introduces a new feature that simplifies the checkout process on its e-commerce platform, and subsequent analysis reveals a significant increase in conversion rates, researchers can infer that the new feature directly caused the improvement.
Establishing Causation:
To establish causation, researchers must demonstrate:
1. Temporal Precedence: The cause must occur before the effect. In our checkout example, the new feature was implemented prior to the observed increase in conversions.
2. Covariation: There must be a demonstrated relationship between the two variables. The data showed that as the feature was adopted, conversions rose.
3. No Alternative Explanations: Researchers must rule out other factors that could influence the outcome. In this case, if marketing efforts or external promotions were also ongoing, researchers would need to ensure that they did not confound the results.
Methods for Establishing Causation
Establishing causation in UX research typically requires more rigorous methodologies than those used for identifying correlations. Here are some key methods:
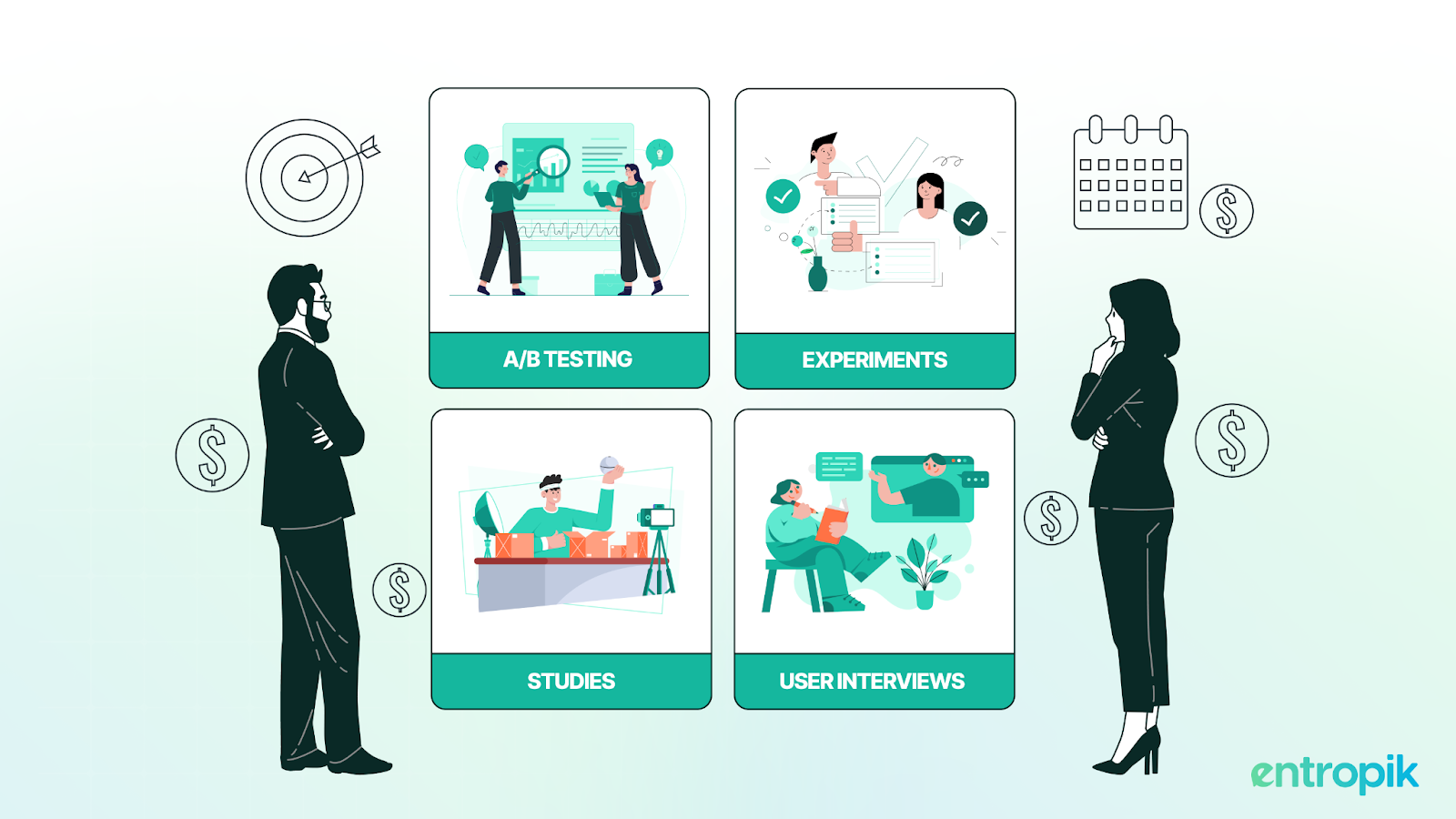
- A/B Testing:
- By randomly assigning users to different versions of a product (Version A vs. Version B), researchers can measure the impact of specific design changes. For example, if a new button design leads to higher click-through rates compared to the old design, this indicates a causal effect.
- Controlled Experiments:
- Controlled experiments involve manipulating one variable while keeping others constant to observe the effects on a dependent variable. For example, a UX researcher may alter the color of a call-to-action button and observe its impact on user clicks, ensuring that other factors (like page content) remain unchanged.
- Longitudinal Studies:
- Longitudinal studies track user behavior over time to establish causal relationships. For instance, a mobile app might implement a new feature and then measure user retention rates over several months to determine if the feature leads to improved long-term engagement.
- User Feedback and Interviews:
- Qualitative methods, such as user feedback and interviews, can provide insights into user motivations and experiences. For example, if users report that a simplified navigation menu made it easier for them to find products, this feedback can help establish a causal link between the redesign and improved user satisfaction.
{{cta-button}}
Practical Application in UX Research
In practical terms, the interplay between correlation and causation can be illustrated through a UX case study.
Let’s say a social media platform notices a correlation between users who receive notifications about friend activities and increased engagement on the site. While this correlation might suggest that notifications boost user engagement, it’s crucial to conduct further tests to establish whether these notifications truly cause an increase in activity.
UX researchers could implement A/B testing, where one group of users receives notifications while another does not. If the group receiving notifications shows a statistically significant increase in engagement, researchers can conclude that notifications have a causal effect on user behaviour. This data not only helps justify design choices but also enables the company to fine-tune its notification strategy for optimal engagement.
Read more: How to conduct Agile UX Research
Conclusion
In summary, the concepts of correlation and causation are fundamental in UX research, guiding researchers in understanding user behavior and informing design decisions. While correlation can highlight interesting relationships and trends, establishing causation provides deeper insights into the direct impacts of design changes. By employing rigorous research methodologies and analytical frameworks, UX teams can effectively distinguish between these concepts, ultimately leading to more informed and impactful user experience strategies. This nuanced understanding empowers organizations to create user-centred designs that resonate with their audience, fostering greater engagement and satisfaction.
This elaboration emphasizes the significance of correlation and causation in UX research, providing a clearer understanding of how each concept applies to real-world scenarios. Let me know if you need further adjustments or additional information!
{{cta-button}}