The mediator vs moderator confusion is not new. When performing research, there has always been some misunderstanding between moderators and mediators. However, these variables significantly differ in statistical aspects, and deciding which is best for you can be challenging.
Incorporating mediators and moderators into your research will help you avoid biases arising from not using them while also enabling you to examine the link between two variables.
Continue reading this article to learn more about the differences between moderators and mediators and their uses in research. So, let’s get started!
What is Mediation in Research?
The first part of the mediator vs moderator debate is understanding what mediation is. Let’s look at what it means-
Mediators give independent factors (also known as intervention or causal variables) a way to influence a dependent variable (also known as an outcome or effect) and illustrate the how and why of the connection between the two. The mediator acts as the "middleman" between independent and dependent variables.
In a mediation analysis, a third variable acts as a mediator or "middleman" between the independent and dependent variables, indirectly allowing the independent variable to infer the dependent variable. Put another way, the causal relationship between the X and Y variables will vanish if the mediator variable is eliminated.
As can be seen in the following image, a mediated model consists of two paths: the indirect effect path (paths B and C) from X → M → Y and the direct effect path between X and Y.

What is Moderation in Research?
The second part of the mediator vs moderator debate is understanding moderation. Let’s look at what it means-
The degree to which the moderator, or a third variable, influences the relationship between the independent and dependent variables is known as moderation analysis. They can influence the variables by either strengthening or weakening the relationship between them.

Mediator vs Moderator Variables
Here is a comprehensive view to help you get more clarity on the mediation vs moderation debate-
Mediating Variables
The effect on the dependent variable by the independent variable is known as a mediator in research. It explains how or why an effect occurs and is a component of the causal pathway of an effect.
Here are a few characteristics of a mediator variable-.
- It has an impact on the dependent variable.
- The statistical correlation between the independent and dependent variables is larger when it is taken into consideration than when it is not.
- By utilizing ANOVAs or linear regression analyses, mediation analysis provides a statistical method for evaluating if a given variable acts as a mediator.
Moderating Variables
The existence, strength, and direction of a relationship between variables are all influenced by moderators. It makes clear to you who, when, and under what conditions a connection will last.
Moderators typically assist you in evaluating the study's external validity by pointing out the boundaries of the instances in which the link between the variables is genuine.
The connection between one dependent variable and two or more independent variables is estimated using multiple linear regression. R programming language can be used for multiple linear regression, and Analysis of Moment Structures (AMOS) can be used for moderation analysis.
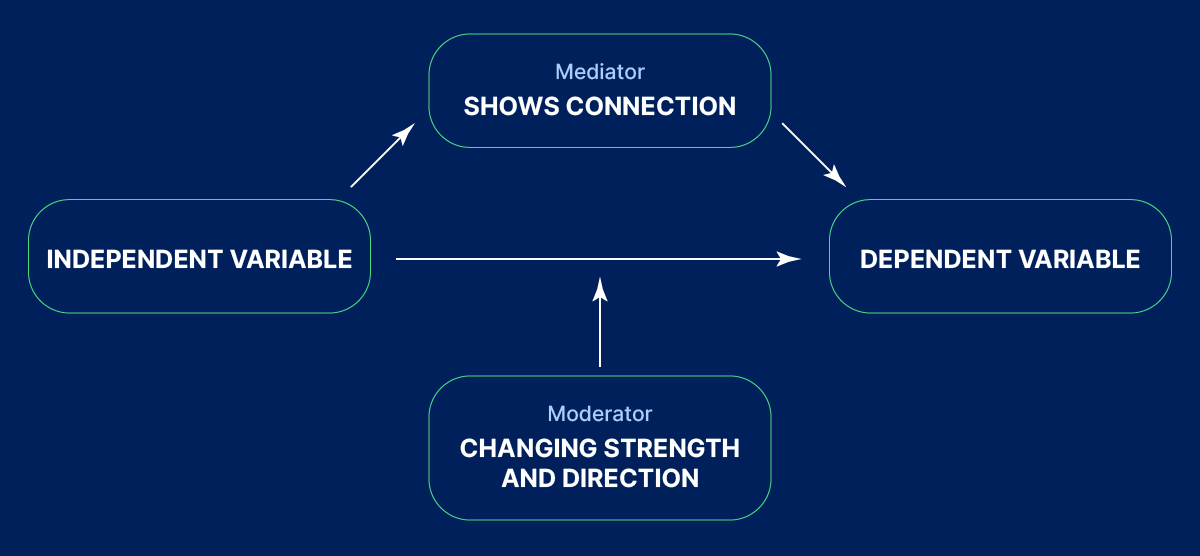
Mediator vs Moderator Examples
Example 1: The relationship between corporate disclosure and gender diversity is influenced by ownership structure.
Here, corporate disclosure is the dependent variable, and gender diversity is the independent variable.
What about the ownership hierarchy? Does an organization's ownership structure directly correlate with its gender diversity? Since ownership structure is not the answer, it needs to be a moderator variable.
Example 2: Social media's impact on loneliness
You make the premise that social media could predict loneliness levels, yet teenagers experience loneliness at a far higher intensity than adults.
Here, the independent variable is the usage of social media, and the dependent variable here is the level of loneliness.
Age here plays the role of a mediator variable as it shows the connection between social media use and the level of loneliness.
{{cta-button}}
{{cta-button}}