Sentiment analysis extracts subjective information from consumer feedback, social media and other important sources. It analyzes the emotion expressed in the text to understand if the data is positive, negative or neutral.
Recent developments in deep learning have greatly improved text analysis algorithms. It is critical to categorize consumer conversations to understand- 1) their top priorities and 2) their fundamental motivations. This combination can accurately analyze millions of consumer conversations and provide in-depth impactful insights.
This article is a comprehensive resource that will help you understand and implement sentiment analysis in your research process. Let’s dive in!
What is Sentiment Analysis?
The technique of extracting and categorizing subjective information or sentiments conveyed in text data is called sentiment analysis, commonly called opinion mining. It entails dissecting and comprehending the feelings, attitudes, and opinions expressed in written content, such as testimonials, news stories, social media posts, and survey results.

Types of Sentiment Analysis
Sentiment analysis primarily concerns a text's polarity (positive, negative, or neutral). Still, it also extends beyond polarity to identify certain moods and emotions (angry, pleased, sad, etc.), urgency (urgent, not urgent), and even intentions (interested vs uninterested).
Here are the most popular types of sentiment analysis used by brands today-
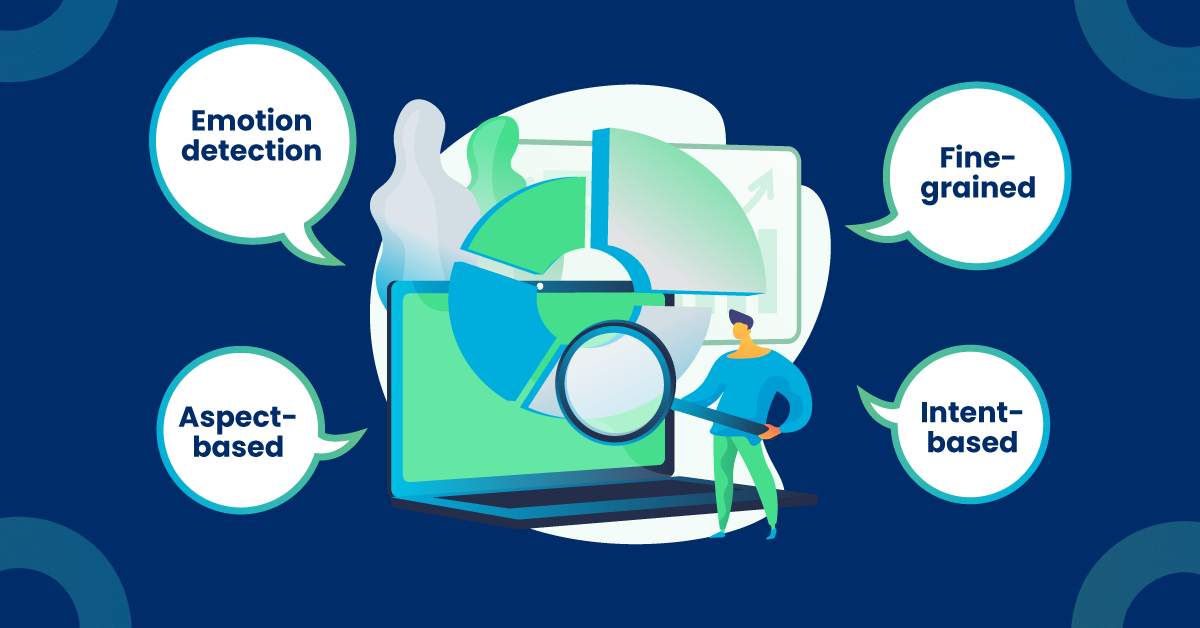
Graded Sentiment Analysis
Graded sentiment analysis, sometimes called fine-grained or continuous sentiment analysis, does more than just classify text as positive, negative, or neutral. It assigns a numerical score or rating to represent the intensity or level of sentiment expressed in the text rather than assigning discrete sentiment categories.
For example-
“The food was fantastic, and the service was outstanding! I recommend trying out this eatery if you’re in New York.”
Sentiment Score: 0.9
Reason: The review uses words like "fantastic" and "outstanding" to convey positive emotions felt by the reviewer towards the eatery. Such significant positive sentiment indicates a sentiment score of 0.9.
Whereas, if the reviewer had mentioned words like “okay”, “slow service”, and “tasteless food”, the sentiment score would range between -0.4 to -0.9.
Emotion Detection Sentiment Analysis
Emotion detection sentiment analysis is concerned with locating and classifying particular emotions represented in the text. It goes beyond typical sentiment analysis by identifying and categorizing emotions like happiness, sorrow, anger, fear, and surprise.
It aims to understand emotional states or the overall sentiment in the text. It helps to learn more about the emotional impact brand initiatives, products, and services create on their target audience.
Learn More: Delivering Empathy-Driven CX with Emotion Insights
Intent-Based Sentiment Analysis
Intent-based sentiment analysis identifies the underlying intent to understand better feelings, motives, or objectives hidden beneath the conveyed expression.
Traditional sentiment analysis only categorizes text as positive, negative, or neutral. Whereas intent-based sentiment analysis dives deeper into answering questions like "Why is the sentiment expressed in this way?" and "What is the purpose behind the sentiment?" in addition to sentiment analysis.
{{cta-button}}
Aspect-Based Sentiment Analysis
It is a specialized method in natural language processing (NLP) concerned with locating and examining the sentiment directed at particular characteristics or aspects of a given subject or entity. It goes beyond conventional sentiment analysis by dissecting the text into minute details and comprehending the sentiment connected to each detail separately.
Aspect-based sentiment analysis aims to extract and classify several features or aspects mentioned in the text before identifying the sentiment connected to each feature.
For example-
“The smartphone's camera is high quality; however, the battery life is disappointing.”
Aspect-based sentiment analysis would recognise "camera quality" and "battery life" as separate aspects and ascertain the related sentiment. It would understand that while opinions on battery life are mixed, opinions on camera quality are generally positive.
Read More: How CMOs Can Utilize Customer Sentiment Analysis to Drive Sales
Why Is Sentiment Analysis Important?
# To Get Actionable Consumer Insights
Sentiment analysis lets brands learn important information about their customers' attitudes, feelings, and preferences.
Brands can learn how customers feel about their products and services by examining the emotion in customer feedback, reviews, social media posts, or polls. This encourages data-driven decision-making and enhances the consumer experience.
# To Monitor and Manage Brand and Reputation
Sentiment analysis enables brands to keep an eye on their online reputation. This helps brands identify negative sentiments and address consumer complaints and grievances.
Brands can maintain or improve their brand image by clearly understanding the consumers' likes and dislikes.
# To Conduct Competitor and Market Analysis
Sentiment analysis plays a vital role in competitor analysis and market research. Analyzing consumer sentiments in feedback, market trends, and social media discussions helps brands identify opportunities from emerging trends.
It also helps evaluate consumers' feelings about your competitors and make informed decisions about your product or services. You can also better position, price, and market your products and services by understanding the market dynamics.
# To Improve Customer Support and Services
Customer care and assistance can be improved using sentiment analysis. Brands can quickly identify and address urgent issues by prioritizing customer comments, support requests, and queries by automatically categorizing them based on sentiment.
Sentiment analysis can also direct customer inquiries to the departments or agents that can best help them, resulting in faster response times, happier customers, and better overall customer service.
{{cta-web}}
How Does Sentiment Analysis Work?

- Preprocessing of Text Data - Unnecessary punctuation is removed, text data is tokenized into individual words or phrases, the text is normalized and stop words are removed.
- Extraction of Features- The text is evaluated for unigrams, bigrams or other linguistic patterns. After this, all relevant features are extracted from the text data.
- Classification of Sentiment- Various machine learning algorithms are used for sentiment classification. These algorithms are trained on datasets where the sentiment for each text data sample is known. In this phase, the algorithms learn patterns and associations between the extracted feature and the sentiment.
- Labeling of Sentiment- After the classifier is trained, it is exposed to identify new, unseen text data. The classifier then tries to identify the sentiment in the text data by assigning sentiment labels based on the training it underwent.
- Aggregation of Sentiment- This is done to analyze larger datasets or monitor trends over a time period.
- Evaluation of Sentiment Analysis Models- The models used to conduct sentiment analysis are optimized to improve their performance. The accuracy of the predictions is measured on a separate sample dataset and adjusted to enhance the accuracy and reduce bias.
Sentiment Analysis Challenges
# Context and Subjectivity
Understanding and interpreting subjective human emotions are necessary for sentiment analysis. It can be difficult to represent the intended meaning when attitudes are very context-dependent effectively. Various people may express similar feelings differently, and cultural or regional differences may make the interpretation more difficult.
# Vagueness and Sarcasm
Textual data frequently includes ambiguous or sarcastic statements, which sentiment analysis models may find challenging to decipher appropriately. Because the literal meaning could be different from the intended sentiment, sarcasm, irony, or complex language can cause misclassification or misinterpretation of sentiment.
# Specificity of Domain
Sentiment analysis methods developed for one area may need help to succeed in another. In diverse contexts, such as social media, product reviews, or financial reports, emotions can be communicated in various ways. To get reliable results, models must be trained or fine-tuned using data from a given domain.
# Handling Contrast and Negation
When negative words are used, the sentiment is often inverted, a common linguistic phenomenon. For instance, the word "not good" conveys a bad feeling. To prevent misclassification, sentiment analysis algorithms should be able to comprehend and handle negations and contrasting statements effectively.
# Multilingualism and Language
Due to nuances, variances, and cultural distinctions specific to each language, sentiment analysis is more difficult in multilingual contexts. Language-specific models or resources may be needed for accurate analysis since different languages may have distinct idiomatic expression patterns, linguistic traits, or patterns for expressing sentiment.
{{cta-button}}
Sentiment Analysis Applications
Sentiment Analysis has a lot of applications across industries and use cases-
- Customer Reviews and Feedback- Sentiment analysis is frequently used to examine customer reviews, ratings, and comments. Brands can learn more about customer happiness, pinpoint areas for development, and better handle consumer complaints. It strengthens brand reputation, optimizing product offers and enhancing customer experience.
- Monitoring Social Media and Brand Reputation Management- Brands can measure brand sentiment, monitor customer sentiment towards marketing efforts, analyze emerging trends, and interact with customers in real time by analyzing feelings expressed in social media posts, comments, and mentions. It enables handling social media platforms efficiently, maintaining brand reputation, and engaging customers proactively.
- Analyze Consumer Conversations- Sentiment analysis analyzes the voice of the customer. Brands learn more about customer happiness, spot reoccurring problems, and enhance customer service operations by compiling and analyzing sentiments expressed in customer interactions, contact centre recordings, and customer support tickets.
And much more!
Conclusion
Sentiment analysis is an NLP method used to examine and ascertain the sentiment conveyed in text data. Preprocessing the text, removing pertinent information, and categorizing the sentiment as positive, negative, or neutral are all steps involved in this process. Sentiment analysis is crucial because it allows for the analysis of markets and competitors, monitor brand reputation, and offers actionable consumer insights.
But, it struggles with issues like subjectivity, context, ambiguity, and data bias. Customer feedback analysis, social media monitoring, brand reputation management, market research, and voice of the customer analysis are some notable applications of sentiment analysis across industries.
{{cta-button}}